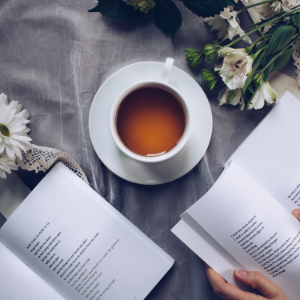
Arxiv Papers
Science & Technology News
Running out of time to catch up with new arXiv papers? We take the most impactful papers and present them as convenient podcasts. If you're a visual learner, we offer these papers in an engaging video format. Our service fills the gap between overly brief paper summaries and time-consuming full paper reads. You gain academic insights in a time-efficient, digestible format. Code behind this work: https://github.com/imelnyk/ArxivPapers
Location:
United States
Genres:
Science & Technology News
Description:
Running out of time to catch up with new arXiv papers? We take the most impactful papers and present them as convenient podcasts. If you're a visual learner, we offer these papers in an engaging video format. Our service fills the gap between overly brief paper summaries and time-consuming full paper reads. You gain academic insights in a time-efficient, digestible format. Code behind this work: https://github.com/imelnyk/ArxivPapers
Language:
English
[QA] Improving the Scaling Laws of Synthetic Data with Deliberate Practice
Duration:00:06:52
Improving the Scaling Laws of Synthetic Data with Deliberate Practice
Duration:00:19:30
[QA] Idiosyncrasies in Large Language Models
Duration:00:07:38
Idiosyncrasies in Large Language Models
Duration:00:20:37
[QA] SigLIP 2: Multilingual Vision-Language Encoders with Improved Semantic Understanding, Localization, and Dense Features
Duration:00:07:36
SigLIP 2: Multilingual Vision-Language Encoders with Improved Semantic Understanding, Localization, and Dense Features
Duration:00:20:03
[QA] Position: Graph Learning Will Lose Relevance Due To Poor Benchmarks
Duration:00:07:31
Position: Graph Learning Will Lose Relevance Due To Poor Benchmarks
Duration:00:27:24
[QA] RocketKV: Accelerating Long-Context LLM Inference via Two-Stage KV Cache Compression
Duration:00:07:37
RocketKV: Accelerating Long-Context LLM Inference via Two-Stage KV Cache Compression
Duration:00:20:26
[QA] Autellix: An Efficient Serving Engine for LLM Agents as General Programs
Duration:00:08:03
Autellix: An Efficient Serving Engine for LLM Agents as General Programs
Duration:00:35:50
[QA] Small Models Struggle to Learn from Strong Reasoners
Duration:00:06:48
Small Models Struggle to Learn from Strong Reasoners
Duration:00:13:23
[QA] TokenSkip: Controllable Chain-of-Thought Compression in LLMs
Duration:00:07:10
TokenSkip: Controllable Chain-of-Thought Compression in LLMs
Duration:00:18:04
[QA] Native Sparse Attention: Hardware-Aligned and Natively Trainable Sparse Attention
Duration:00:07:40
Native Sparse Attention: Hardware-Aligned and Natively Trainable Sparse Attention
Duration:00:26:01
[QA] (How) Can Transformers Predict Pseudo-Random Numbers?
Duration:00:07:57
(How) Can Transformers Predict Pseudo-Random Numbers?
Duration:00:21:33