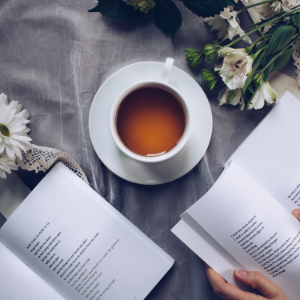
Arxiv Papers
Science & Technology News
Running out of time to catch up with new arXiv papers? We take the most impactful papers and present them as convenient podcasts. If you're a visual learner, we offer these papers in an engaging video format. Our service fills the gap between overly brief paper summaries and time-consuming full paper reads. You gain academic insights in a time-efficient, digestible format. Code behind this work: https://github.com/imelnyk/ArxivPapers
Location:
United States
Genres:
Science & Technology News
Description:
Running out of time to catch up with new arXiv papers? We take the most impactful papers and present them as convenient podcasts. If you're a visual learner, we offer these papers in an engaging video format. Our service fills the gap between overly brief paper summaries and time-consuming full paper reads. You gain academic insights in a time-efficient, digestible format. Code behind this work: https://github.com/imelnyk/ArxivPapers
Language:
English
[QA] Nemotron-H: A Family of Accurate and Efficient Hybrid Mamba-Transformer Models
Duration:00:08:11
Nemotron-H: A Family of Accurate and Efficient Hybrid Mamba-Transformer Models
Duration:00:25:17
[QA] Agentic Knowledgeable Self-awareness
Duration:00:07:13
Agentic Knowledgeable Self-awareness
Duration:00:18:40
[QA] Inference-Time Scaling for Generalist Reward Modeling
Duration:00:08:07
Inference-Time Scaling for Generalist Reward Modeling
Duration:00:18:00
[QA] Multi-Token Attention
Duration:00:08:04
Multi-Token Attention
Duration:00:18:18
[QA] Visual Jenga: Discovering Object Dependencies via Counterfactual Inpainting
Duration:00:06:59
Visual Jenga: Discovering Object Dependencies via Counterfactual Inpainting
Duration:00:16:15
[QA] Wan: Open and Advanced Large-Scale Video Generative Models
Duration:00:08:19
Wan: Open and Advanced Large-Scale Video Generative Models
Duration:01:04:43
[QA] UI-R1: Enhancing Action Prediction of GUI Agents by Reinforcement Learning
Duration:00:08:31
UI-R1: Enhancing Action Prediction of GUI Agents by Reinforcement Learning
Duration:00:16:39
[QA] SWI: Speaking with Intent in Large Language Models
Duration:00:07:45
SWI: Speaking with Intent in Large Language Models
Duration:00:09:45
[QA] Unified Multimodal Discrete Diffusion
Duration:00:07:16
Unified Multimodal Discrete Diffusion
Duration:00:20:09
[QA] Self-Supervised Learning of Motion Concepts by Optimizing Counterfactuals
Duration:00:17:51
Self-Supervised Learning of Motion Concepts by Optimizing Counterfactuals
Duration:00:17:51